Introduction
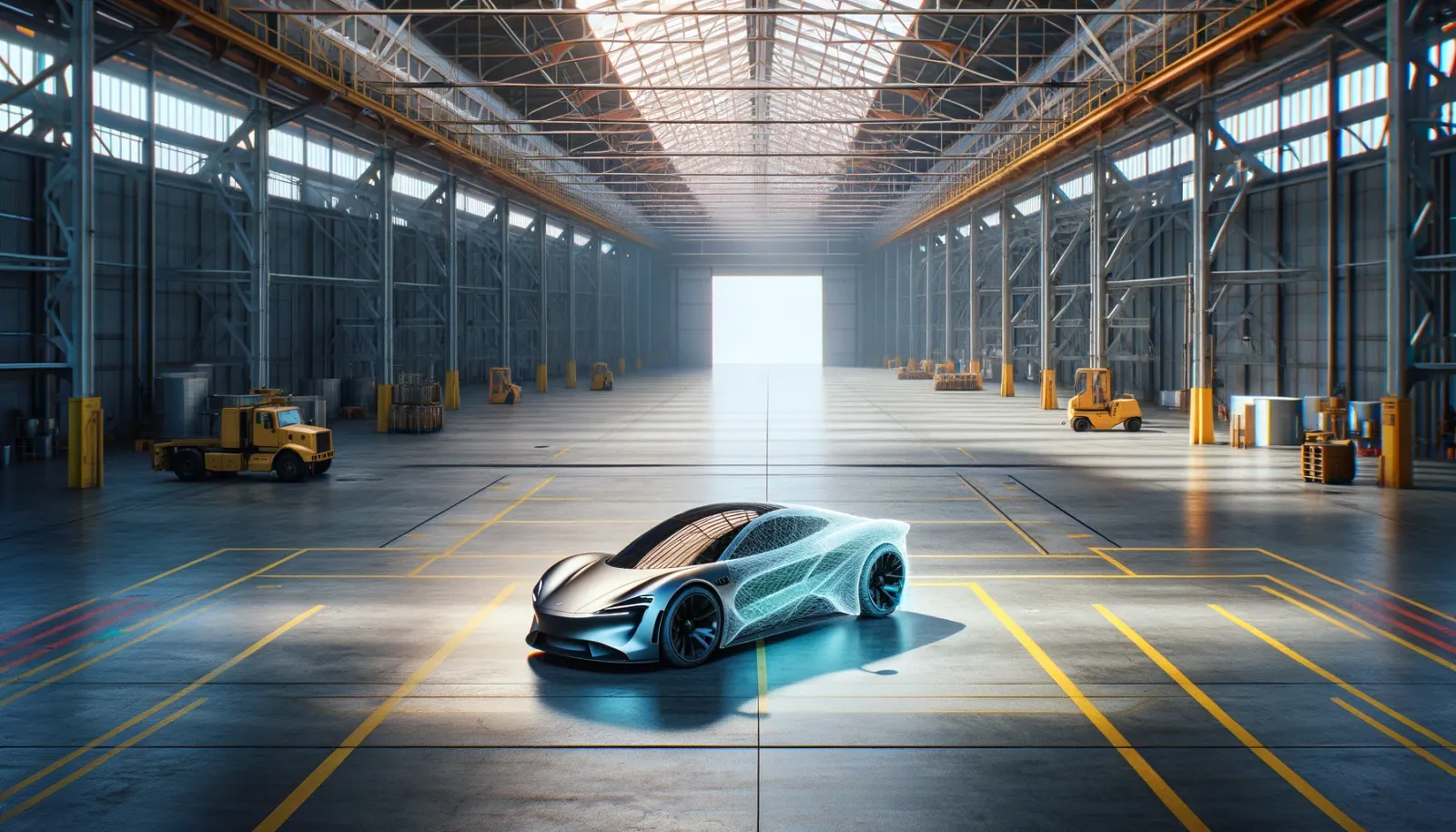
The increasing presence of Digital Twins in the automotive industry signals a major shift, promising to transform the landscape of vehicle design, development, and maintenance. But do we understand Digital Twin concept in the same way? In this article, we will discuss what a Digital Twin is, what it does, and why it's used.
Another important question is whether the implementation of this technology in the automotive industry meets the evolving demands of consumers. In this article, we will explore how the use of Digital Twins enables the pragmatic resolution of complex problems faced by car manufacturers, thereby meeting consumer demands for the next generation of vehicles.
Fundamental Concept of Digital Twin
So, what is a Digital Twin? Adhering to the commonly accepted definition, Digital Twin is a data-driven virtual model that accurately mirrors a physical object, system, or process. Based on the twin, it is possible to model, predict, and provide insights across various scenarios of design, enhancement, production, and maintenance of its physical counterpart. I agree that it's not the most unambiguous definition =) But I promise, by the end of this article, we will have a clear understanding of what a Digital Twin is.
Let's take a brief look into the history of the Digital Twin. Originating from a need for better product lifecycle management (PLM), Michael Grieves introduced the concept in 2002. The PLM model aims to create real and virtual spaces to store information for both domains. Later in 2010 NASA’s John Vickers popularized this concept and coined the term Digital Twin. In fact, at this point, NASA has been using virtual replicas of spacecraft and aircraft for years to study or simulate the real systems. This technology has since transcended beyond its initial aerospace applications, embedding itself into various sectors, such as the automotive industry, where it plays a pivotal role in enhancing vehicle design, manufacturing, and maintenance processes.
The basic use case scenario for the Digital Twin technology implies that the data exchanged between the physical and virtual parts creates a cycle – that is, the data from the physical object helps improve the accuracy of its Digital Twin, and the data obtained from analyzing the twin's behavior under various conditions helps optimize the physical object. When the digital copy is updated with the relevant data, the virtual model may be used for the implementation of various simulations, which can lead to potential improvements, by creating valuable information that can then be applied back to the original system existing in the physical world.
Of course, if Digital Twin was always used in such a straightforward way, there’d be little doubt in its definition and application. However, the Digital Twin technology extends far beyond simple scenarios. In fact, organizations can use this technology in many ways depending on the industry they operate in, what stage of the product lifecycle they are at, what they are trying to model and optimize, who or what the Digital Twin is for, and much more.
Digital Twin in Automotive Industry
In the automotive industry, Digital Twins are most commonly virtual replicas of the entire car, its software, mechanics, electrics, and physical behavior. These Digital Twins maintain real-time data on performance, sensors, and inspections, as well as service history, configuration changes, parts replacement, and warranty information. A pioneer of this approach in the automotive industry is Tesla, which has created virtual replicas of every car sold. Data from thousands of cars' sensors, applications, and even superchargers continuously flow into each car's simulation at the factory. This enables them to monitor whether the car is functioning properly or requires further maintenance.
As I mentioned earlier, in automotive manufacturing, the first Digital Twins were created by digitizing or copying real vehicles. However, thanks to technological progress, Digital Twins are now created before their physical originals. The term 'twin' is misleading because, in essence, a Digital Twin can represent a compilation of all the digital and physical models of a physical object that does not yet exist. A physical copy that will come into existence once the models reach a certain level of maturity. For example, Renault described how they implemented the Digital Twin technology in mid-2022. They create a virtual model at the design stage and conduct various tests before creating a physical twin. After the physical twin is manufactured and sold, feedback data based on real-world usage is fed back to its Digital Twin. This allows them to optimize their workflow and reduce the time taken to design a new vehicle from an entire year to just one quarter.
But the potential of this technology goes beyond merely creating digital replicas of physical objects. It enables you to simulate and test the performance of your design, production processes, and much more. This technology allows for predictive analysis and scenario testing. As the automotive sector aims to meet increasing demands, Digital Twins prove to be invaluable. For instance, in a collaborative study between SODA and VSOptima, Digital Twins of the development processes in various automotive companies, which utilize different methodologies and tools, were created. This study identified bottlenecks and potential improvements. Companies that embrace a feature-driven development approach and use the SODA SDV Kit demonstrated a twofold productivity gain!
Digital Twins have now become a fundamental part of the automotive industry. This has revolutionized how people involved in vehicle design, manufacturing, and service actually work. Design, manufacturing, maintenance, customer service: all have improved output, and can "interact" with each other through the car's Digital twin, providing and consuming data in real-time throughout the car's lifespan.
But is it always practical to use Digital Twins? After all, Digital Twin is a model filled with large volumes of data, having a complex data structure, and the modeling process itself sometimes requires a huge number of model experiments. Defining such a mathematical model manually is always a very labor-intensive process, and sometimes simply unfeasible. Here, Artificial Intelligence-based modeling tools come to our aid. The ability of Artificial Intelligence (AI) to efficiently process and analyze large data sets, understand complex data relationships, and generate model experiments ensures that Digital Twin modeling is not only feasible but also accurate and scalable.
The use of AI for solving this set of tasks has become virtually indispensable. It could even be said that the use of Digital Twins has opened up opportunities for AI to interpret Software-Defined Vehicles!
Requirements, Design and Implementation
The use of the Digital Twin technology in the design and development phases in the automotive industry marks a transformative shift towards more efficient, innovative, and cost-effective vehicle production. At the heart of this revolution lies the ability to simulate, test, and validate vehicles and their components in a virtual environment long before a physical prototype is ever built. This capability not only accelerates the design process but also opens up a new realm of possibilities for enhancing vehicle performance and safety.
The creation of a Digital Twin occurs already at the stage of requirements elicitation and analysis, because such high-level requirements define the attributes of the designed vehicle. Subsequently, the propagation of vehicle-level attributes, according to the mathematical model of the Digital Twin, affects the final configuration of parameters of internal components of the car. The Digital Twin acts as a living mathematical model, constantly refined through updates with data about the behavior, construction, and optimization of its physical counterpart.
Virtual prototyping, facilitated by Digital Twins, allows for the detailed examination of how vehicle components interact under diverse scenarios. This approach also allows architects and engineers to model and optimize the performance of vehicles across various parameters, such as engine efficiency, aerodynamics, and fuel consumption. These capabilities ensure that the final product meets safety standards and performance requirements, thereby effectively responding to consumer demand for next-generation vehicles.
I would also like to draw your attention to a not-so-obvious advantage provided by the use of Digital Twins - the collaborative way of working. Because in reality, during the design and implementation process, highly detailed and realistic models are created, which allow for better visualization of the developing vehicles for all participants in the process. Such Digital Twin offers a single source of truth for all parties involved in the project: from designers to contractors and stakeholders. By providing access to identical information in real-time, it ensures better communication, collaboration, and decision-making throughout the development process. This leads to increased efficiency, reduced errors, and enhanced transparency, as all stakeholders are on the same page, working towards a common goal.
The Digital Twin technology is a powerful tool for designing and developing next-generation vehicles. Thanks to virtual replicas, the industry can achieve a higher level of innovation, efficiency, and quality, making data-driven design decisions.
Verification and Validation
The era has already passed when OEM manufacturers relied exclusively on fleets of test vehicles and Hardware-in-the-loop (HIL) testing. These traditional methods, although effective, are increasingly viewed as cumbersome and resource-intensive. Today, the emphasis has shifted towards Digital Twin testing, allowing most tests to be conducted virtually. The complexity of modern automotive systems, burdened with heterogeneous embedded systems running on multiple operating systems, requires a more flexible and iterative development process. Digital twins make this possible, reducing the need for physical prototypes and laboratory equipment and enabling quick evaluation of multiple design variations. Also Digital Twins is particularly effective when validating a physical prototype is unrealistic. Sometimes prototypes are too expensive, operate in conditions that are difficult to replicate, or require human intervention. In these scenarios, using a Digital Twin instead of its physical counterpart can help you understand the behavior of your product with significantly less investment.
Autonomous vehicles need to navigate a wide range of situations and make decisions in fractions of a second. Testing these scenarios in the real world can be both complex and risky. Given the need to comply with the SOTIF standard for autonomous vehicles, the automakers face the need for a reliable and scalable way to automatically create unknown/unsafe scenarios and to quantitatively assess the criticality of such scenarios. These scenarios include extreme cases that cannot be anticipated using a requirements-based approach. And here, once again, the Digital Twin technology comes to our aid. After all, nothing prevents us from making the surrounding test environment also part of a Digital Twin and using a simulation-based testing approach regarding the obtained digital model. Thus, engineers gain a safe virtual environment in which they can simulate complex scenarios and traffic conditions using Digital Twins.
Of course, there remains the issue of a tool in which one could perform simulations based on the obtained digital models. Also, we are most likely interested in the ability to automatically generate unknown/unsafe scenarios and the ability to quantitatively assess the criticality of such scenarios. Having such a tool would allow for confidence in the completeness of test coverage. SODA provides such a tool - SODA Sim, which automates the creation of testing scenarios thanks to the use of AI in generative modeling the conditions of the surrounding test environment and supports the simulative modeling of the Digital Twin's behavior in the conditions of this environment.
Also, it's important to note the potential of using a Digital Twin as a virtual "sandbox" for testing the deployment of new functionalities on vehicles in operation. The ability to continuously test software updates within the Digital Twin environment not only accelerates the deployment process but also enhances the quality of the products. For instance, a Digital Twin can verify the software update process, ensuring its reliability and the absence of disruptions in the operation of existing functionalities before deploying it on real SDVs. This level of preliminary testing minimizes risks and leads to a more optimized and reliable deployment process.
As a result, digital models can completely replace the physical prototype at the testing stage; they can also undergo virtual crash tests, virtual driving scenarios, "digital" wind tunnel tests, and more. Each simulation is performed much faster and in far greater quantities than physical tests could ever be. With the complete digitization of the design and creation of a vehicle, virtual tests can be conducted two years before the actual tests. It's easy to understand the progress that Digital Twin technology represents.
Post-Production Maintenance and Evolution
In the ever-evolving automotive industry, the adoption of the Digital Twin technology during the operational phase of vehicles presents next pivotal opportunities: enhancing customer service, predictive maintenance, reducing costs, and improving vehicle performance and safety over its lifecycle. Digital Twins allow for the continuous monitoring and early detection of potential issues, facilitating proactive maintenance and minimizing downtime.
Predictive maintenance uses Digital Twins for continuous monitoring and forecasting the condition of physical vehicles in real time. These predictions are made based on the analysis of data collected from sensors on the vehicles, which are continuously fed into their Digital Twins. Based on such a Digital Twin, the behavior of a vehicle is modeled under various conditions to detect potential malfunctions before they occur. The predictive capabilities are based on complex data analysis and modeling methods using AI, which can perform real-time simulation modeling to accurately forecast potential failures, wear, and the remaining service life of vehicle components. This approach enables companies to schedule maintenance only when necessary, reducing downtime and extending the vehicle's lifetime.
Leveraging Digital Twins for performance optimization in operational vehicles offers a significant advancement in how automakers manage and enhance vehicle functionality. By utilizing real-time data and advanced analytics, these virtual replicas can pinpoint inefficiencies and suggest optimizations for improved performance and safety. One example of performance optimization could be improved battery management to extend its lifespan. The use of AI-based solutions, working with the Digital Twin, allows for adjustments to changes in the battery's condition by monitoring charge-discharge cycles.
As such, Digital Twins not only elevate the driving experience but also offer a strategic advantage in maintaining and updating vehicles efficiently, ensuring they meet the dynamic demands of modern consumers and regulatory standards.
Conclusion
In conclusion, the adoption of the Digital Twin technology in the automotive industry marks a transformative era, enabling manufacturers to meet the growing demands of modern consumers. As Digital Twins continue to evolve, their role in the automotive sector will undoubtedly increase, leading universally to the only correct way of creating and servicing vehicles based on their virtual replicas. Of course, automakers must possess a set of tools that can operate Digital Twins; without them, the use of this technology is impossible. SODA offers a complete set of such tools - SODA SDV Kit for the design, implementation, and validation of vehicles based on their Digital Twins.